The (so-called) Quantitative-Qualitative Divide
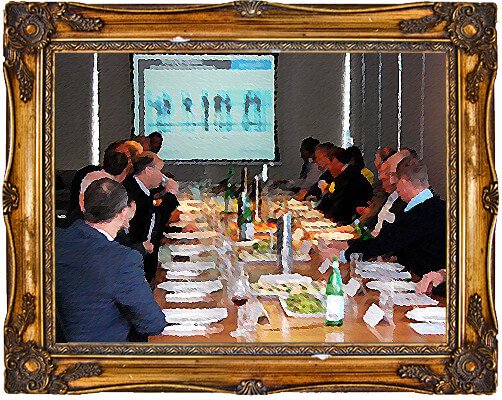
Having A Picnic
Users and consumers of products, services, and systems experience these across cultural, social, psychological, and physical dimensions. Accordingly, there are a wide variety of researchers and research methods employed by business – ethnography itself includes a host of methods in its toolkit. A broad diversity of methods and epistemologies is necessary because, as Nafus and Anderson, citing Knorr-Cetina, point out, “‘science’ is not a unified entity, and to locate epistemologies in social arrangements is to expose the fiction of a singular scientific method” (2006:245).
However, Sunderland has observed that the commodification of research in business conforms around different priorities. “Corporations have developed systems of pre-qualifying research ‘suppliers’ based on the cost of distinct research methods and abstracted from any real research question at hand (e.g., the cost of an in-home interview, a focus group, a diary)” (Sunderland 2013:132). Ken Riopelle contends that “a company’s typical request for proposal… often specifies the research questions, methods, and timing the responding vendor, contractor, or supplier should use in a research study… [and] an internal company project manager or team, who may or may not have expertise in research methods, commonly reviews and approves a contractor’s instruments and methods” (Riopelle 2013:41). In fact, in her article on epicpeople.org, “The Automation of Qualitative Methods,” Sally A. Applin explains that for many of those who are “not steeped in research, [or who] lack a research degree… data collection is their definition for research, rather than methods merely being a part of what research encompasses” (2017).
From a business perspective, integrating research within normal business processes allows them to better predict the cost, timing, and resources to allocate to research projects. However, determining an appropriate research design through an initial discovery phase ensures that their questions can be answered and their objectives can be met by the proposed research methods. In her article, “The Problem With Only Looking At Big Data,” Carol Rossi writes that a discovery phase is absolutely necessary “otherwise at best you won’t have the full picture, or worse, will draw incorrect conclusions” (2016). It is vital for practitioners to convey the importance of matching the appropriate research method or methods to the questions and objectives of the study tasked by a business to ensure that credible answers, insights, and conclusions can be drawn from the data.
The Allure of Big Data From A Business Perspective
One area of particular methodological contention is that between analytics and ethnography in the so-called quantitative-qualitative divide. With the advent and proliferation of digital technologies into nearly every facet of life today there is almost no research topic in the modern world that doesn’t include a virtual or technological component. Many of these new technologies also record large amounts of user-data – either on the back-end or as a function of its purpose – that can then be aggregated and analyzed. Armed with these data mining tools, “practitioners of analytics emphasize the fact that they have a much larger robust data set that can generate true behavioral insights” (Maxwell 2013:176).
While these practices raise ethical issues concerning privacy and power, the notion that vast amounts of data can be automatically and immediately collected with little cost or training is an alluring proposition for many businesses. The promise of big data and analytics has also generated considerable methodological contentions as a competing alternative with ethnography and other forms of qualitative research. As Julia Gluesing observes, “many ethnographers as well as their employers are beginning to raise strong arguments for dropping the labor-intensive, time-consuming, and costly conventional ethnographic methods in favor of electronic tools” (2013:23).
However, the same concerns regarding what can be logically inferred from the data and the suitability of the method to the questions being asked and the targeted objectives still persists with analytics as with any other research methodologies. In his chapter contribution, “Accelerated Pattern Recognition, Ethnography, and the Era of Big Data,” in the book, Advancing Ethnography in Corporate Environments, Chad R. Maxwell points out that “analytics is often focused on reporting, prediction, and modeling, but the human implications and meanings are lost on the audience” (2013:186).
Bridging The Quantitative-Qualitative Divide
Still, there is an unavoidable implication in our modern, technologically-driven world that traditional ethnographic methods alone are no longer sufficient for understanding networked communications and virtual practices. These new realities greatly expand the scope of what needs to be observed and studied. Many fields that involve a digital or virtual component require technology-supported methods of data collection and forms of observation in order to gain an understanding of the whole picture.
However, practices within digital and virtual realms are all still culturally-inflected, socially negotiated, and even locally contextualized. In their introduction to the book Digital Anthropology, Horst and Miller point out that, “no one lives an entirely digital life and… we cannot easily treat each new media independently since they form part of a [still wider world that both impacts upon and transcends that frame]… we can only understand practice within the much wider context of more traditional extended ethnography” (2012:16). As a reflection of these new realities, students and early-career practitioners looking to work in business would do well to include a mixed bag of traditional and technology-oriented research methods into their repertoire.
The Anthropology of Big Data
An important issue with analytics and Big Data best raised by Maxwell is the concern that “analytics often happens in a black box, offering up a response without context or clear transparency around the algorithmic rules that computed a judgment, answer, or decision” (2013:182). While analytics and data mining tools are designed and marketed as a cheap and easy-to-use solution for understanding the world, the often proprietary technology being developed and the algorithmic rules governing them are becoming more and more complex and cryptic to its users (see Maxwell 2013). Instead of augmenting their user’s ability to make sense of the world, the danger is that the assumptions and values algorithmically embedded within analytic software, tools, and systems unduly impact its users’ interpretations and decisions without them being fully aware of it.
Citing Lombardi, Marietta L. Baba notes that “indeed, this process would be a questionable practice in anthropology; data torn from context means the value of interpretation declines even if the ‘business proposition’ appears reasonable on the surface” (2014:57). Maxwell argues that because analytics are complex, man-made systems “we must question the rules, powers, and judgments that govern it, the inherent values and biases the human-created algorithms contain, and the users who implement and craft the analytics that make the decisions” (2013:184). There is an opportunity, and even a need, for practicing anthropologists to play a strategic role in the development and critical analysis of analytics and those that craft them to ensure that “it doesn’t completely supplant human judgment, logic, and intuition” (Maxwell 2013:184, also see Nafus 2014).
Conclusion
Dominique Desjeux’s framing of the capabilities of the different scales of observation is helpful in understanding the distinctions between big data and ethnography that in many respects make them complementary research methodologies. Suggesting that “what is visible on one scale can become invisible or change shape at another level of observation . . . and the interest of the scales is to shift focus to bring out contextual effects that vary from one scale to another” (2014:105). Along with the unit of analysis, Neal Patel also points to the heuristic differences between quantitative and qualitative research that are in no way oppositional to each other but that together can present a clearer picture of reality. “Qualitative research concerns itself with understanding and decoding subjective, phenomenological (symbolic, mental, gestural) content because it regards these as the structure of social order. Quantitative research conscientiously limits itself to what is objectively (tangibly, materially) measurable, because it cannot confidently treat ‘subjective’ content” (2014:144).
By merging together accounts from both scales and leveraging the particular qualities of analytics and ethnography, a more comprehensive picture can be formed that will allow for a better understanding of the subject area. Apart from playing a strategic role in the development and critical analysis of analytics, ethnography can help analytics “contextualize their work using human and cultural frameworks” (Maxwell 2013:186). While analytics can help ethnographers to better manage and aggregate data, as well as to analyze and hone in on much larger global phenomena.
Works Cited
Applin, Sally A. 2017. “The Automation of Qualitative Methods.” EPIC. https://www.epicpeople.org/automation-qualitative-methods/ (accessed March 23, 2017).
Baba, Marietta L. 2014. “De-Anthropologizing Ethnography: A Historical Perspective on the Commodification of Ethnography as a Business Service.” In Handbook of Anthropology in Business, edited by R. Denny and P. Sunderland, 43-68. New York, London: Routledge.
Desjeux, Dominique. 2014. “Professional Anthropology & Training in France.” In Handbook of Anthropology in Business, edited by R. Denny and P. Sunderland, 100-116. New York, London: Routledge.
Gluesing, Julia. 2013. “Being There: The Power of Conventional Ethnographic Methods.” In Advancing Ethnography in Corporate Environments, edited by B. Jordan, 23-37. Walnut Creek, CA: Left Coast Press.
Horst, Heather A. and Daniel Miller. 2012. “The Digital and the Human: A Prospectus for Digital Anthropology.” In Digital Anthropology, edited by H.A. Horst and D. Miller, 3-38. London, New York: Berg.
Maxwell, Chad R. 2013. “Accelerating Pattern Recognition, Ethnography, and the Era of Big Data.” In Advancing Ethnography in Corporate Environments, edited by B. Jordan, 175-192. Walnut Creek, CA: Left Coast Press.
Nafus, Dawn, and Ken Anderson. 2006. “The Real Problem: Rhetorics of Knowing in Corporate Ethnographic Research.” Proceedings of the 2nd Ethnographic Praxis in Industry Conference 2006:244-258.
Nafus, Dawn. 2014. “Making Markets Emerge: Enumeration, ‘Development,’ and Technology Markets.” In Handbook of Anthropology in Business, edited by R. Denny and P. Sunderland, 167-185. New York, London: Routledge.
Patel, Neal H. 2014. “Methodological Rebellion: Overcoming the Quantitative-Qualitative Divide.” In Handbook of Anthropology in Business, edited by R. Denny and P. Sunderland, 135-156. New York, London: Routledge.
Riopelle, Ken. 2013. “Being There: The Power of Technology-Based Methods.” In Advancing Ethnography in Corporate Environments, edited by B. Jordan, 38-55. Walnut Creek, CA: Left Coast Press.
Rossi, Carol. 2016. “The Problem With Only Looking At Big Data.” Medium. https://medium.com/@crossiUX/the-problem-with-looking-at-only-big-data-808e25f87ff6#.orydh53y0 (accessed March 28, 2017).
Sunderland, Patricia. 2013. “The Cry for More Theory.” In Advancing Ethnography in Corporate Environments, edited by B. Jordan, 122-35. Walnut Creek, CA: Left Coast Press.
Recommend0 recommendationsPublished in Big Data, Business Anthropology, Digital Anthropology, Employer Resources, Quantitative Research
I find it interesting how the dominant culture frames research data as Quantitative & ~Quantitative (not Quantitative). Qualitative from that framework is thus defined as anything that is not Quantitative. In extreme cases (having worked in Data Science & AI), “qualitative” means “not numbers”. Whereas in spaces I’ve occupied with other non-quantitative researchers across various disciplines, “Qualitative” was understood as “what I do” & there was an assumption that everyone else does it that way too. This tends to happen when we are forced into a “non-quantitative” space. People fixated on the quantitative modality leave little room for any other way of seeing. My favorite discussion of this is D. Neyland’s Ethnography of Numbers (https://onlinelibrary.wiley.com/doi/abs/10.1002/9781118325513.ch10). I summed up his perspective on counting in research as follows: 1) Accounting (we count things that are countable); 2) Recounting (we count things that we can count again); 3) Discounting (we don’t count things we can’t count). Here’s a presentation I gave at O’Reilly OSCON on my take on what “Computational Anthropology” could be like – https://www.youtube.com/watch?v=akn9l7m4j_E